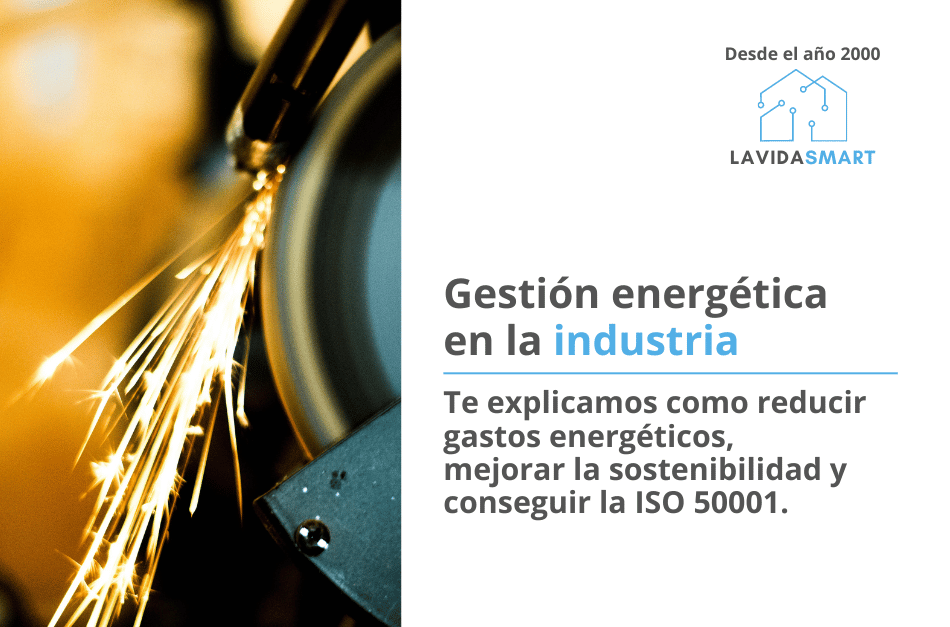
Presentación de Gestión Energética para la Industria
Hemos preparado una presentación dedicada a un aspecto vital para la sostenibilidad y la eficiencia
In the landscape of contemporary discussions, energy consumption and Artificial Intelligence (AI) have emerged as transformative forces, reshaping our sustainability approach and reducing energy consumption. Beyond transient trends, they embody a powerful synergy that holds the potential to redefine our energy future. This article delves into the intrinsic connection between these concepts and explores how cutting-edge technologies contribute to a more efficient tomorrow. Additionally, the concluding segment sheds light on the symbiotic relationship between AI and energy storage, underscoring how this pairing is poised to propel us towards even more efficient energy grid.
As our energy demands surge, so does the emission of greenhouse gases. Simultaneously, Machine Learning undergoes continuous refinement, revealing improved techniques and algorithms. This evolutionary journey unfolds a promising vision for a more efficient and sustainable future. Businesses and organizations are actively venturing into creative applications of Machine Learning and AI in the energy sector, with a specific focus on predicting energy consumption and optimizing the performance of renewable sources.
Synonymous with Automated Learning, Machine Learning empowers computer programs to autonomously enhance performance through experiential learning. These programs leverage meticulously crafted algorithms and statistical models refined over years of research. In the energy sector, these datasets are fed into Machine Learning algorithms, effectively «training» them. An exemplary embodiment of this integration is the lavidaSMART Energy Management Platform, which seamlessly combines Machine Learning and AI to predict energy consumption accurately.
Energy consumption often remains unchanged until unforeseen events, like soaring electricity bills or renewable energy underperformance, capture our attention. It is at these junctures that machine learning and artificial intelligence come into play. One key application involves predicting energy consumption using deep learning and regression analysis. Analyzing historical energy consumption data unveils trends and patterns, facilitating precise predictions of future consumption.
Transforming energy consumption into monetary metrics goes beyond simple bill estimation; it serves as a cornerstone for businesses and individuals to make strategic and informed decisions. By assigning a monetary value to energy consumption, entities gain a comprehensive understanding of the financial implications associated with their energy usage. This not only facilitates budgeting but also empowers organizations to identify cost-saving opportunities, optimize resource allocation, and strategically invest in energy-efficient technologies. In essence, economic value becomes a catalyst for intelligent, financially sound decisions that align with sustainability goals and overall operational efficiency.
The realm of energy consumption extends beyond numerical data; it involves the nuanced understanding of «how» and «why» energy is utilized. Practical insights derived from comprehending these aspects become instrumental in driving informed habit changes. This goes beyond a mere quantitative assessment and delves into the qualitative realm of energy behavior. Individuals and businesses can leverage this understanding to cultivate conscious energy habits without compromising productivity or quality of life. Practical insights become a catalyst for a sustainable shift in behavior, fostering a culture of energy consciousness and responsible consumption.
At the core of effective energy management lies technical precision, achieved through enhanced data management practices. This involves meticulous data collection and analysis methodologies that form the bedrock for accurate predictions. Technical precision ensures that the insights derived from energy data are reliable, actionable, and reflective of the intricate dynamics of energy consumption patterns. The integration of advanced technologies and methodologies elevates the predictive capabilities of energy models, allowing businesses and individuals to proactively respond to fluctuations, optimize efficiency, and navigate the evolving landscape of energy needs with confidence. In essence, technical precision becomes the linchpin for orchestrating a sophisticated and responsive energy management strategy.
Energy consumption prediction contributes to operational efficiency by allowing businesses and individuals to streamline their energy usage. With accurate forecasts, organizations can optimize their operations, ensuring that energy resources are allocated efficiently. This results in cost savings, reduced wastage, and an overall improvement in operational productivity.
Energy consumption prediction is undergoing a paradigm shift, driven by an array of innovative technologies within Artificial Intelligence (AI) and Machine Learning (ML). These advancements reshape our ability to forecast and optimize energy usage. Let’s explore these cutting-edge innovations propelling effective energy consumption prediction.
One of the foundational innovations in this landscape is Time Series Forecasting. By introducing data at regular intervals, this method empowers us to predict energy consumption patterns accurately. This is particularly vital for understanding and adapting to the dynamic nature of energy usage, especially in the context of renewable energy sources.
Artificial Neural Networks, a subset of Machine Learning incorporating deep learning algorithms, play a pivotal role in processing massive datasets efficiently. As the complexity of energy data continues to grow, these advanced algorithms enhance our capacity to analyze historical data, providing valuable insights into consumption trends.
Ensemble Methods, a collective of techniques that combine predictions from various models, elevate prediction accuracy and robustness. By integrating results from decision trees, support vector machines, and neural networks, these methods mitigate biases and errors inherent in individual models. This collaborative approach is particularly impactful in deciphering complex and dynamic energy consumption patterns.
A significant leap in innovation involves incorporating external factors into predictive models. Holidays, industrial activities, events, and political changes can influence energy consumption patterns. By accounting for these external dynamics, predictive capabilities are significantly improved, allowing for more accurate and adaptable energy consumption forecasts.
Transfer Learning, a concept within Machine Learning, leverages knowledge acquired in one domain to streamline the training of new prediction models in a related domain. This innovation reduces the time and resources required for training new models from scratch. Particularly useful in scenarios with limited data availability or transitions between regions with distinct consumption patterns, it enhances the adaptability of prediction algorithms.
The dynamic nature of energy consumption demands real-time adjustments in predictive models. Online Learning addresses this need by continuously updating models based on new data. This ensures that prediction models adapt swiftly to changes in consumption patterns, providing accurate insights into evolving energy needs.
As AI and ML become integral to energy consumption prediction, the need for transparency and interpretability becomes paramount. Explainable AI methods offer insights into how models arrive at specific predictions. This transparency builds trust among stakeholders, fostering confidence in the predictive process. Particularly crucial in industries with stringent regulatory requirements, explainable AI ensures that decisions are well-founded and understandable.
A notable addition to the arsenal of innovations is Predictive Maintenance applied to energy systems. By employing AI algorithms to predict when equipment is likely to fail, organizations can proactively schedule maintenance, preventing costly breakdowns and ensuring optimal energy efficiency. This not only reduces downtime but also contributes to substantial energy savings.
Incorporating these innovations into energy consumption prediction not only refines prediction accuracy but also expands the scope of AI and ML applications in the energy sector. This continuous evolution holds the potential to redefine energy management practices, contributing to a more sustainable and efficient energy future.
While machine learning addresses practical, economic, and environmental aspects, the integration of advanced energy storage systems becomes a crucial innovation in the realm of renewable energy sources—embracing solar, wind, rain, geothermal and other solutions. These sources, while clean and environmentally friendly, pose challenges due to their inherent unpredictability. Energy storage solutions act as a buffer, mitigating the impact of intermittent energy production and enhancing overall grid efficiency and reliability. Let’s delve into the multifaceted contributions of these systems that go beyond conventional stabilizing functions.
Energy storage systems, more than mere stabilizers, form the bedrock of a reliable and consistent energy supply. Operating as dynamic reservoirs, they intelligently store surplus energy during periods of heightened production. This stored energy is judiciously released during low production phases, creating a seamless and stable energy flow. This not only reduces dependency on external factors, such as unpredictable weather conditions, but also ensures a continuous and uninterrupted power supply. In essence, energy storage systems act as architects of energy stability, laying the foundation for a resilient and unwavering power grid.
Beyond mere smoothing, energy storage solutions excel in mitigating the inherent intermittency of renewable energy sources. By addressing variations in energy production, these systems guarantee a steady and predictable flow of energy to the grid. The ability to modulate energy release in real-time minimizes disruptions caused by fluctuations in renewable energy generation. This predictability is paramount for maintaining grid stability and meeting the ever-evolving demands of consumers, industries, and the overall energy ecosystem.
The integration of energy storage augments the flexibility of the energy grid, propelling it into a realm of enhanced adaptability. This newfound flexibility allows for more adept management of energy demand and supply. The grid becomes responsive, adapting to sudden shifts in energy consumption patterns with agility. This adaptability is crucial in navigating the complexities of a modern energy landscape, where dynamic changes in demand and the integration of diverse energy sources require a grid that can flex and accommodate seamlessly.
Efficient prediction, synergistically coupled with energy storage, orchestrates an optimized transition between renewable and non-renewable sources. This strategic balancing act ensures not only the most cost-effective but also the environmentally sustainable energy mix. The dynamic interplay between predictive insights and stored energy empowers decision-makers to make real-time adjustments, fine-tuning the energy transition to align with economic and ecological goals. It marks a paradigm shift towards a harmonious coexistence of various energy sources, guided by data-driven precision.
Beyond acting as a backup, energy storage becomes a robust shield, fortifying the overall resilience of the energy system. In the face of unexpected disruptions, whether due to sudden weather changes or unforeseen technical issues, stored energy serves as a reliable buffer. This resilience ensures a continuous and reliable power supply, preventing downtime and offering a safety net during critical moments. It exemplifies how energy storage systems are integral components in building a robust and adaptive energy infrastructure.
Energy storage systems transcend their conventional roles, emerging as dynamic collaborators in shaping a future where efficient prediction, sustainability, and resilience converge for a more reliable and sustainable energy ecosystem.
In the nexus of energy consumption and Artificial Intelligence, a potent synergy emerges, reshaping our sustainability paradigm. This article explored how AI, especially in Machine Learning, drives accurate energy predictions and optimization. Advantages include Economic Value, Practical Insights, and Technical Precision, fostering informed decisions and sustainable habits.
Innovations like Time Series Forecasting and Ensemble Methods refine predictions, while the alliance of AI with energy storage systems stabilizes supply, mitigates intermittency, and enhances grid flexibility. Together, they propel us towards a resilient, efficient, and sustainable energy future.
Contact us for further information.
Hemos preparado una presentación dedicada a un aspecto vital para la sostenibilidad y la eficiencia
Hemos preparado una presentación dedicada a un aspecto vital para la sostenibilidad y la eficiencia
Domótica integrada con equipos de máxima seguridad en mansión de lujo
Nos encantaría escucharte. Si tienes preguntas sobre nuestros productos y servicios o deseas más información, no dudes en ponerte en contacto con nosotros.